From mimicking to critical thinking
The history of language models starts in 1883 with the concept of semantics, developed by the French philologist, Michel Bréal — founder of modern semantics. He studied the ways languages are organised and how words are connected within a language.
Natural language processing (NLP) gained great popularity after the end of World War II in 1945. People realised through peace talks that translating automatically from one language to another is crucial.
Active research on NLP started with machine translation projects such as the Georgetown-IBM experiment (1954). IBM's Arthur Samuel created a computer checker-playing program. In 1959, he developed algorithms that enabled his program to improve, calling this “machine learning.”
In 1958, Frank Rosenblatt combined Hebbian learning with Samuel's work on machine learning to create the first artificial neural network (ANN), known as the Mark 1 Perceptron.
ELIZA (1966), was the first computer program in the world — an early natural-language processing computer program that could conduct human-like conversations. ELIZA could recognise simple user inputs and respond based on pre-defined scripts, using methodologies like pattern-matching recognition, and substitution methodology that gave users an illusion of understanding. An outstanding feature from ELIZA was that it showed emotions and empathy, for example:
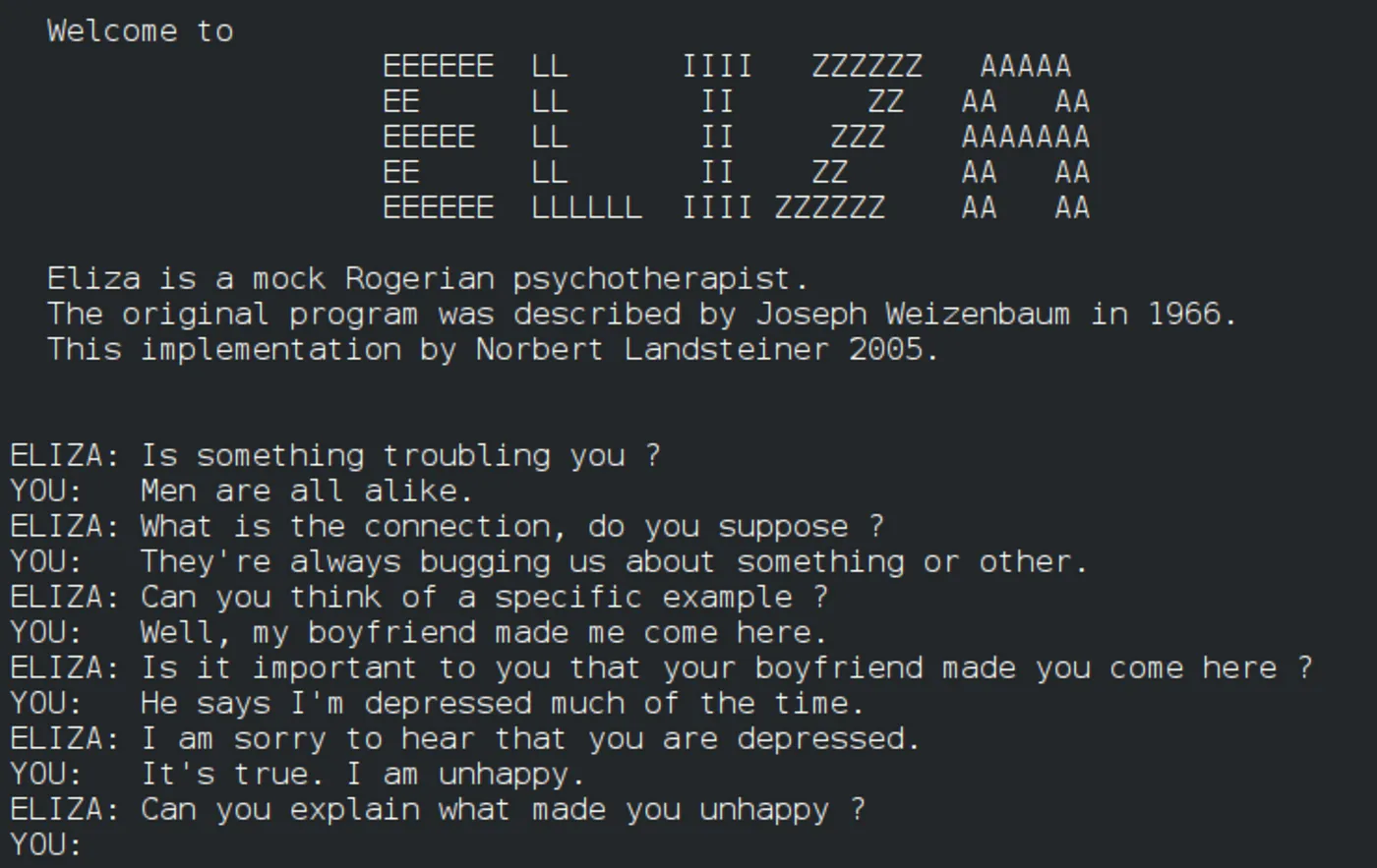
Conversation with ELIZA. Source Wikipedia.
Let's jump to the 90s era, where text analysis and speech generation methods like N-Grams and Recurrent Neural Networks became very popular. In 2006, Google Translate was launched as a multilingual neural machine translation service. It was able to translate text, documents and websites from one language to another.
Apple's Siri, was the first successful NLP/ AI assistant in the world in 2011. Siri's automated speech recognition module translates the user's words into digitally interpreted concepts. The voice-command system then correlates those concepts to predefined commands and performs specific actions. For example:
Siri Task 📝 — Answering Call
Siri Command 🤖 — “Hey Siri, answer the phone”
But Siri had many problems recognising and interpreting user commands, especially in the presence of accents, dialects, or noisy environments.
In 2017, the Transformer architecture was introduced by researchers at Google. This model incorporates self-attention mechanisms to capture dependencies and relationships within input sequences and to improve previous architectures for machine translations.
The Transformer model led to the development of pre-trained systems, such as Generative Pre-trained Transformers (GPT) and Bidirectional Encoder Representations from Transformers (BERT).
The first major breakthrough in text generation was with GPT-2 in 2019, which could generate semantic sentences. Then, in 2020 came another big jump with GPT-3, trained in much the same way as GPT-2 but with roughly an order of magnitude (i.e., 100x) more parameters.
In 2022, OpenAI released ChatGPT. ChatGPT significantly surpasses GPT-3 in a range of tasks, including communicating in human-like English, developing new software, and writing speeches.
Alongside the release of GPT-3's, Google released T5 (Text-to-Text Transfer Transformer). T5 is a transformer-based that uses a text-to-text approach (where both the input and output are text strings).
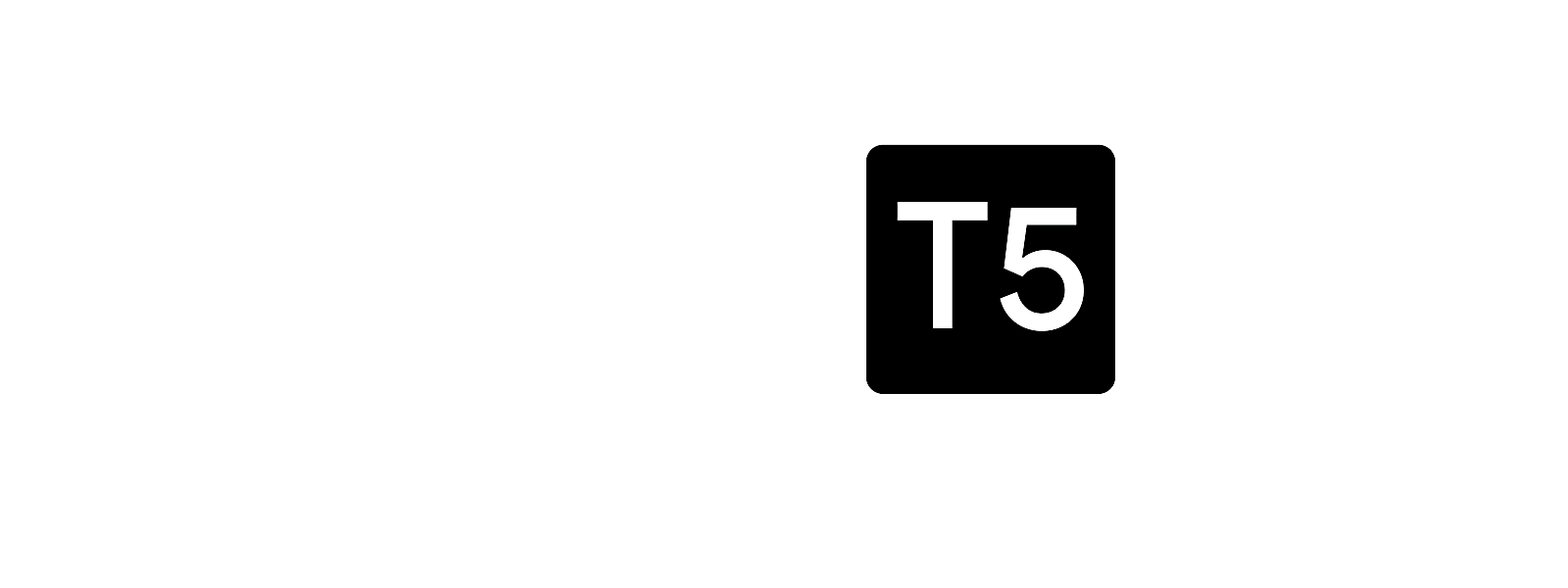
Diagram of text-to-text framework. Image from https://research.google/blog/exploring-transfer-learning-with-t5-the-text-to-text-transfer-transformer/.
OpenAI's GPT-4 marks a new milestone in the evolution of LLMs. GPT-4 is a multimodal large language model that incorporates three main capabilities: creativity, visual input, and longer texts. These capabilities allow for deeper contextual understanding, and multi-step reasoning setting the basis to introduce critical thinking.
OpenAI states that GPT-4 is
“More reliable, creative, and able to handle much more nuanced instructions than GPT-3.5.”
For example:
User 🙎🏻♂️ — “Write an essay comparing the economic policies of the UK and the US.”
LLM 🤖 — “Sure. Would you like the comparison to focus on their historical impact, theoretical differences, or real-world applications?”
Despite these capabilities, GPT-4 still makes foolish mistakes and produces false statements. GPT-4, for instance, has been revealed to have a good grasp of algorithms but struggles with arithmetic or notation.
Still GPT-4 performs poorly in critical reasoning. In his research paper, M. M. Jahani Yekta, explains that this is possible because the training data does not generally include domain logic (thinking process leading to solutions) and the limitations of the next-word-prediction architectural paradigm.